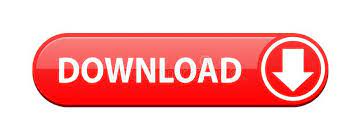
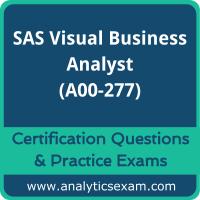
Integrate open source languages and agile technology with the capabilities of SAS analytics.

SAS provides application and enterprise developers, data scientists, and analysts with access to SAS services. Chapter 9, “Process Considerations for Data Quality,” finally shows the process aspect of data quality and also discusses aspects like data relevancy and possible alternatives.Open Source SAS Open source development resources for developers.Chapter 8, “Analytics for Data Quality,” shows additional requirements of interdependences of analytical methods and the data.Chapter 7, “Predictive Modeling,” discusses special requirements of predictive modeling methods.Chapter 6, “Data Correctness,” checks whether the available data are correct with respect to its definition.Chapter 5, “Data Completeness,” deals with the fact that available data fields may contain missing values.Chapter 4, “Data Quantity,” examines whether the amount of data are sufficient for the analysis.Chapter 3, “Data Availability,” starts with the question as to whether data are available in general.The data quality criteria that are defined in this book in chapters 3–9 are the following. Consequences of Poor Data Quality-Simulation Studies.The naming and ordering of these three parts and the respective chapters follow a process as well, but also consider a segmentation of the content of this book into well-defined parts and a good readable sequence of topics and chapters. This book is divided into three main parts.
#HOW TO DOWNLOAD SAS VISUAL ANALYTICS SERIES#
Simulation studies and consequences of poor data quality for predictive modeling and time series forecasting.
#HOW TO DOWNLOAD SAS VISUAL ANALYTICS HOW TO#
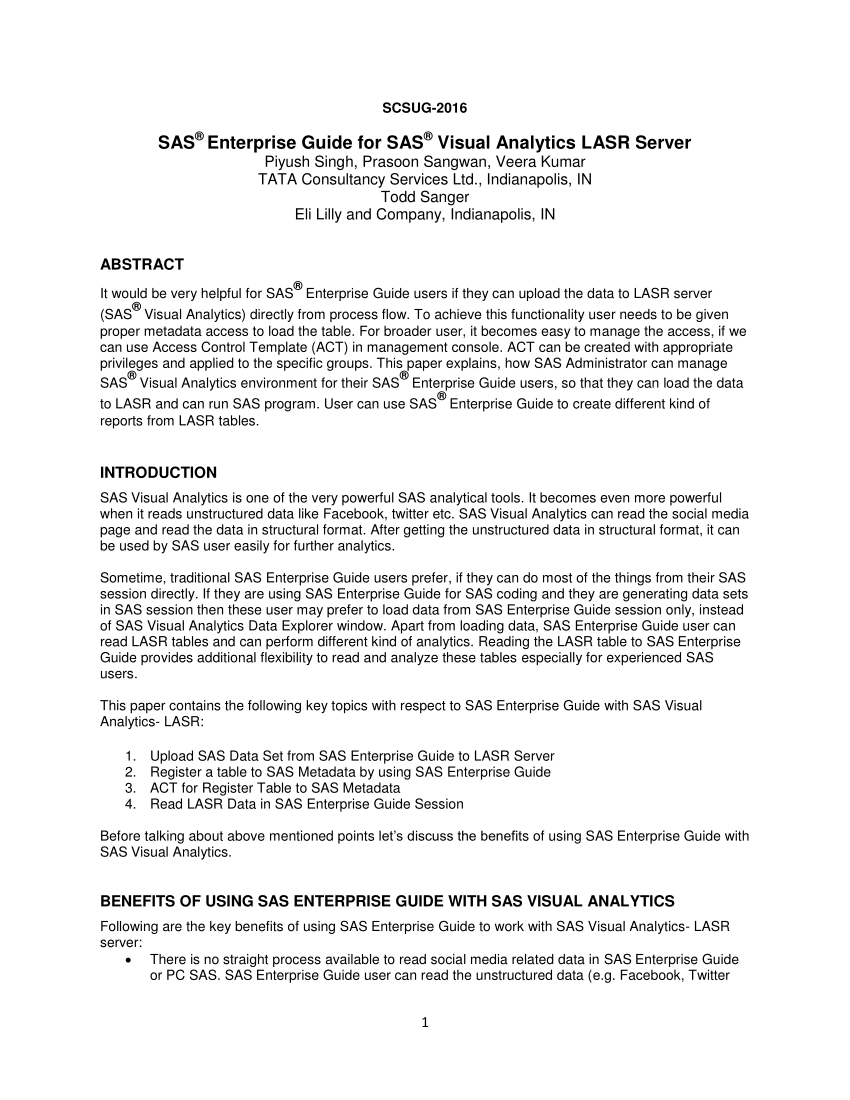
It will not be said here that these topics are not important for analytics on the contrary, they build the foundation of data for analysis. However, most of the initiatives, publications, and papers on data quality focus on classical data quality topics, such as elimination of duplicates, standardization of data, lists of values, value ranges, and plausibility checks. My intention was not to write another book on data quality in general, but to write the first book that deals with data quality from the viewpoint of a data scientist, statistician, data miner, engineer, operations researcher, or other analytically minded problem-solver.ĭata quality is getting a lot of attention in the market. Main focus of this book -> Analytics and Data Science Other books from Gerhard Svolba at SAS Press.ĭata Preparation for Data Science Using SASĪpplying Data Science - Business Case Studies Using SAS “Rosetta Stone” - The most important text sample in history and the role of labeled data in machine learning
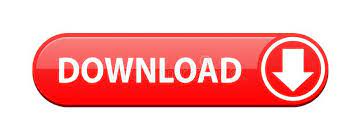